Genomic and Structural Big Data to Identify Cancer Drivers
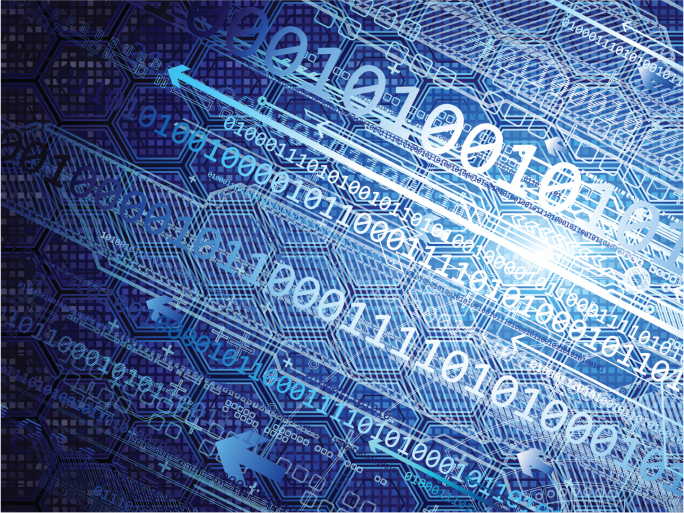
A research team lead by the Sanford Burnham Prebys Medical Discovery Institute (SBP) has identified more than 100 new cancer drivers using the power of Big Data. The researchers combined the information of two libraries containing genomic and structural data to determine mutations that alter protein-protein interactions and lead to cancer. It is the first time that protein 3D structure is used to predict cancer. The study presents an algorithm that takes into account the specific location of the mutations in the genes and how the different protein structures obtained will interact with the same drug. The result was published in the journal PLOS Computational Biology.
The age of internet and the exponential progress of the information technology has brought an unprecedented amount of shareable data repositories. A fairly new discipline, called Big Data, focuses on extracting the relevant material from the vast pool of information, identifying patterns and generating useable knowledge. In biology, the arrival of Big Data is paticularly important, with multiple -omics libraries at disposition, constructed collaboratively with the data generated by thousands of researchers around the world. Importantly for the cancer field, genomic libraries contain useful mutation data, and pharmacological datasets have been crossed with the former to generate insightful conclusions. However, all studies so far identify only the mutated genes, and not the particular mutations in those genes. This is a major flaw, given that the specific location of a mutation can translate in totally different phenotypes depending on the affected protein region. Proteins are modular micromachines, and drugs act in a specific protein domain, be it an interaction domain or a catalyst domain. By not taking into account what part of the protein is being affected by a mutation, datasets might be assigning a pharmacological phenotype to the wrong mutation.
An algorithm that identifies protein domain importance in drug sensitivity
The authors created an algorithm, e-Drug, to identify specific protein locations that, when altered, change the sensitivity to a particular drug. e-Drug integrates data from 6000 patients affected by tumors with 18000 protein structures. Genomic and structural information was retrieved from The Cancer Genome Atlas and the Protein Data Bank. The algorithm analyzes whether alterations in protein structure are enriched in cancer mutations, thus identifying cancer drivers.
The new algorithm will help explain why mutations in the same gene have different patient outcomes and drug response. Using data from patients and cancer cell lines, molecular mechanisms of drug effectiveness are resolved, and patient response and survival can be predicted.